Operating on a patient thousands of miles away, exploring faraway planets, safely disposing of hazardous materials—these previously impossible or dangerous tasks are now achievable through teleoperation, the process of remote controlling robots. However, successful teleoperation requires proper training.
Since human operators come from diverse backgrounds and have varying levels of robotics experience and spatial planning abilities, one-size-fits-all training is ineffective. To address this, a team of Johns Hopkins researchers has developed a targeted training approach that achieves consistent performance across users, regardless of their inherent ability to visualize objects and actions in 3D.
The team presented its findings at the 2024 IEEE/RSJ International Conference on Intelligent Robots and Systems, held October 14–18 in Abu Dhabi, U.A.E.
“Our method ensures that everyone, regardless of innate ability or experience with robots, can accurately operate a robot remotely,” says co-lead author and Malone Fellow Tsung-Chi Lin. “Our personalized approach levels the playing field and has the potential to make this technology more accessible to and reliable with a wider range of applications.”
Joining Lin on this study are Juo-Tung Chen, a master’s student in robotics and the other co-lead author of the paper, and Chien-Ming Huang, a John C. Malone Assistant Professor of Computer Science.
Traditional teleoperation training has relied on two limited approaches: either users self-identifying their skill gaps or working directly with a small number of expert trainers. In contrast, the Hopkins team’s approach consists of two simple steps: first, assessing users’ natural abilities, then providing training modules tailored to their individual skill gaps.
The team’s system evaluates users’ abilities in three key areas essential for remote manipulation tasks: moving and rotating a robotic arm, positioning the arm within a specific space, and controlling the arm’s gripper to grasp and release objects. Then, it matches users to training modules that focus specifically on the areas in which they need improvement.
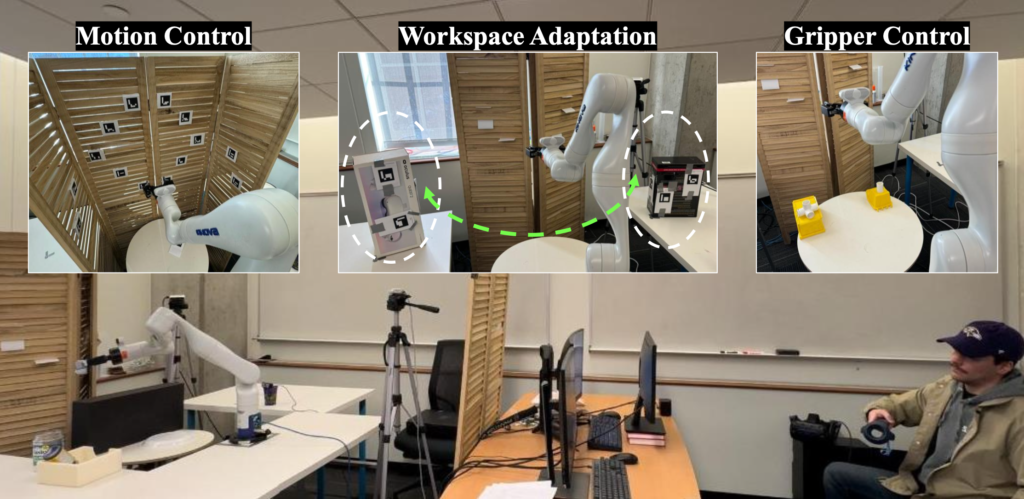
The team’s proposed targeted training paradigm includes three distinct modules: robot motion control, workspace adaptation, and gripper control.
The researchers tested their approach by having study participants operate a robotic arm to prepare medications remotely. Compared to free-form training, the team’s targeted training method demonstrated a more consistent performance, with users reporting higher confidence levels.
“Our results also suggest that our method reduces the impact of natural spatial ability on task completion time and performance,” says Lin.
This means that anyone—regardless of their innate ability to visualize a robot’s movements in 3D—can benefit from this targeted training approach.
“In practical terms, this could improve the reliability and safety of teleoperation in high-stakes environments like health care or hazardous material handling,” says Lin. “Remote-controlled critical tasks, such as those in surgery or disaster response, could be performed more effectively—regardless of the operator’s inherent spatial skills.”
The researchers plan to adapt their training method for use with more complex robots. They also aim to investigate this method’s long-term skill retention to inform the development of more comprehensive teleoperation training programs.