Explainability—the ability of an artificial intelligence model to present its reasoning clearly so that both doctors and patients can understand its decisions—is widely considered a crucial consideration for AI systems being integrated into real-world health care practice. But depending on their design, different approaches to increasing the transparency behind an AI’s decision-making can also lead clinicians to over- or under-trust the AI, which may be ineffective—or even dangerous—for patients.
To find the right balance between explainability and trust, a team of Johns Hopkins clinicians and computer scientists conducted an experiment in which 220 doctors analyzed chest X-rays with simulated AI assistance. The researchers found that more specific AI explanations had mixed effects: While increasing physicians’ diagnostic accuracy and efficiency, they also fostered misplaced trust even when the AI’s recommendations were wrong.
Their work appears in Radiology.
“Our findings show how explainable AI can be a double-edged sword,” says co-author John C. Malone Associate Professor of Computer Science Suchi Saria. “Specific explanations helped doctors make faster and more accurate diagnoses, but that transparency also increases the risk of over-trust. The challenge now is to design AI systems that are transparent enough to support clinical decision-making while still encouraging a healthy amount of skepticism, when warranted.”
For the study, the Hopkins team—which was also led by John C. Malone Associate Professor of Computer Science Chien-Ming Huang and computer science PhD students Drew Prinster and Amama Mahmood—asked a group of radiologists and internal or emergency medicine physicians to review eight chest X-rays.
During their review, the doctors were assisted by “ChestAId,” a simulated AI assistant designed to match the diagnostic performance of expert radiologists in interpreting chest X-rays. The researchers manipulated the AI tool’s accuracy, confidence levels, and the type of explanation it provided to see how these factors affected the physicians’ diagnostic performance and perception of and trust in the AI.
The researchers evaluated two types of AI explanations. The first, local explanations, explain why a specific prediction was made based on a particular input; in this instance, these explanations pointed out unusual or important areas on the X-ray that influenced the AI’s decision, similar to how a doctor might circle concerning spots on an image. The other type, global explanations, described how the AI tool functioned in general, demonstrating how its decisions were made by comparing the selected X-ray with a similar one from the data on which the AI was originally trained.
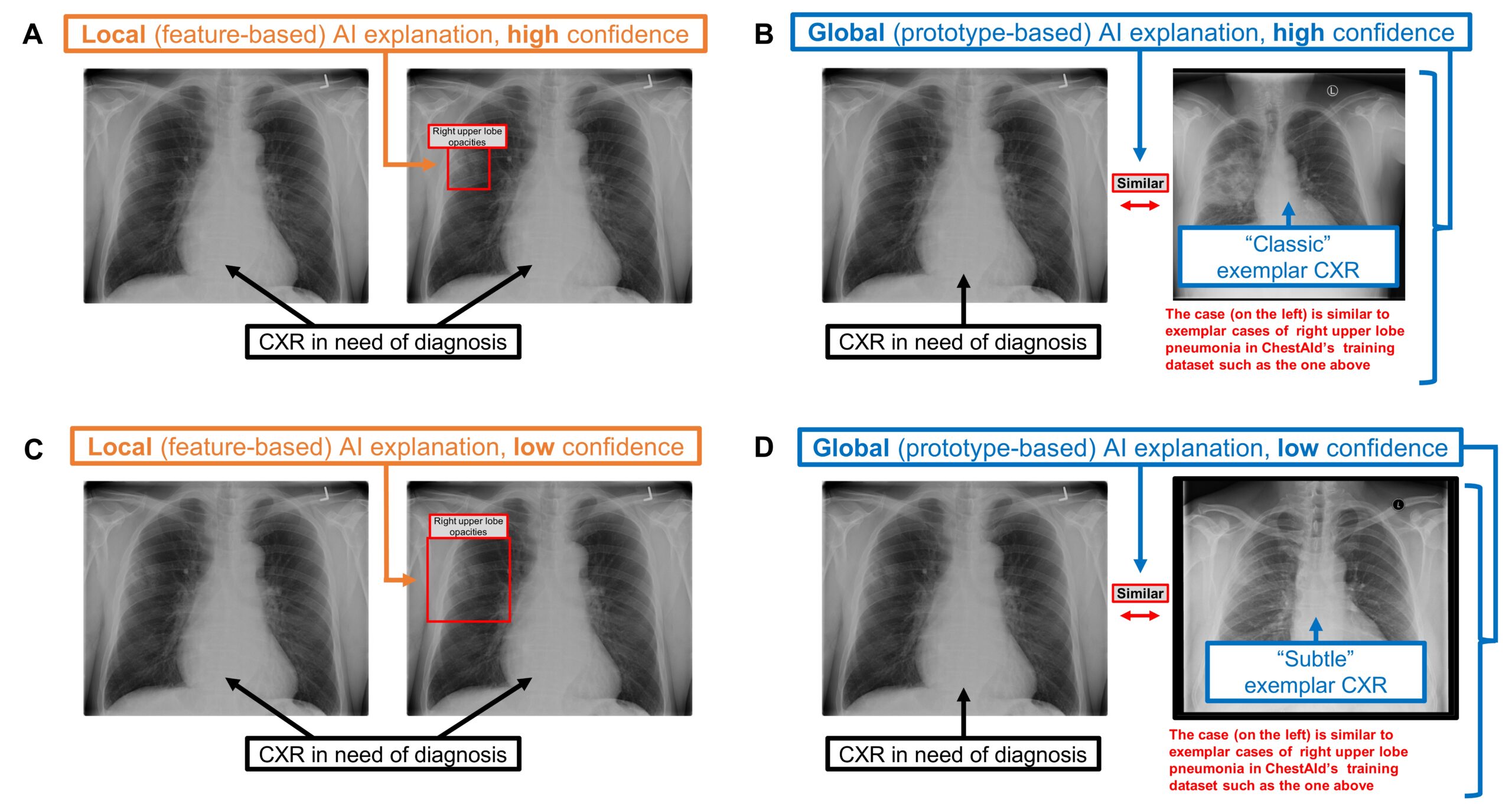
Chest radiograph examples of local vs. global AI explanations.
Regardless of the type of explanation offered, the doctors could make one of the three responses to the AI’s suggestion: 1) accept it, 2) keep parts of it but modify others, or 3) reject it completely and complete the diagnosis task on their own. After selecting their response, the doctors finalized their diagnosis, reported their confidence in their findings, and rated how useful the AI was in helping them complete the task.
“Our results demonstrated that the type of AI explanation affected the physicians’ diagnostic performance—even if the physicians themselves may not consciously realize such effects,” says study first author Prinster. “AI developers and stakeholders need to keep this in mind when designing systems for effective human-AI teaming.”
Specifically, local explanations helped physicians make more accurate diagnoses when the AI’s advice was correct. These explanations also took the physicians less time to review, making the diagnosis process more efficient, the team reports.
The researchers also measured “simple trust,” or reliance without verification—how quickly a user accepts (or disagrees with) AI advice without verifying it. They found that the participating physicians were more likely to place simple trust in an AI that provided local explanations, regardless of whether its advice was correct to begin with.
“This is likely the reason we saw the local AI explanations improve diagnostic accuracy and efficiency when the advice was correct,” says Prinster. “However, when AI advice is wrong, local explanations may lead to overreliance on and misplaced trust in errors, which should be explored further in future work.”
The team recommends that future efforts to develop AI decision support systems carefully consider the complex influences of design elements, paying particular attention to the unique effects of various explanation types.
“A promising approach might be to consider a dynamic combination of AI explanations—both local and global—with calibrated AI uncertainty to promote appropriate trust in AI advice,” says Prinster.
“It’s clear that AI will be playing a big part in all aspects of everyday life,” says Saria. “Figuring out how AI can best communicate with its human counterparts will have a widespread impact on the future of how we work.”
Additional authors of this work include Paul Yi, an adjunct assistant research scientist in the Malone Center; Cheng Ting Lin, an associate professor of radiology and radiological science at the Johns Hopkins University School of Medicine; and Jean Jeudy, a professor of diagnostic radiology and nuclear medicine at the University of Maryland School of Medicine.
This work was supported by the National Science Foundation.