BY JIM DUFFY // PHOTOS BY WILL KIRK
There’s treasure in all those tweets and Google searches we send out into cyberspace each day, says Mark Dredze. He is leading high-tech tracking efforts that could yield important insights on everything from drug overdoses to suicide prevention.
The internet went haywire when the news broke about Charlie Sheen on that Tuesday morning in November 2015. In the days after the actor revealed live on television’s Today show that he had HIV, the virus that causes AIDS, millions of people ventured online to share the news and see what everybody else had to say about it.
For most people, mornings like that have become part and parcel of day-to-day life in the 21st century, with its celebrity-driven news cycles and ubiquitous access to social media. Facebook feeding frenzies along the lines of Sheen’s announcement rise up and then fade away with increasing regularity. The phenomenon has grown only more intense now that the country has a president who sends Twitter off the rails every time he twitches his thumbs.
Mark Dredze’s experience of social media is different. The Whiting School of Engineering associate professor has spent most of the past decade working at the leading edge of a fast-developing young field called social monitoring. Here, the goal is to sort through the gigantic haystack of our collective online activity in search of nuggets of scientific insight that can boost our understanding of human behavior and help inform public policy decisions.
Dredze’s focus is public health, so it was natural that the Sheen news would land on his to-do list. In fact, it presented an opportunity to dispel a key doubt harbored by skeptics of social monitoring. That doubt is this: Do the words people type on their keyboards bear a reliable relationship to the things that happen in their so-called real lives?
“Everyone in marketing bought into the idea that this connection is real 10 or 15 years ago, right? That’s why we see all those ads when we’re online,” says Dredze, the John C. Malone Associate Professor in Computer Science and visiting professor at the Applied Physics Laboratory. “But for a lot of people in other fields—and especially in public health, it seems—this can still be kind of a new idea.”
The primary tools of the trade in social monitoring are machine learning and natural language processing. When Dredze put them to work on search engine data from the time around Sheen’s announcement, he found, as expected, that activity on the topics of HIV and AIDS had indeed skyrocketed. Interestingly, he also found that the level of that activity dwarfed numbers that had been drummed up over the years by public awareness campaigns along the lines of World AIDS Day.
But it was the next step in Dredze’s work that drove the extensive media coverage about his findings. Digging a little deeper into the search engine data, he found a surge of interest in information about getting tested for HIV and, especially, about home testing kits. Then he went to the companies that make those kits and asked for sales data and trend lines.
“Let’s just say it became very clear that a lot of the people who went searching for information about getting tested for HIV also went ahead and took the next step of buying a kit,” Dredze says. “It was a pretty powerful confirmation that there really is that relationship between what people do online and what they do offline.”
COMING OF AGE
Dredze found his way to social monitoring eight years ago during a round of small talk with one of his graduate students, Michael Paul, PhD ’15. The two were wrapping up a long day at a conference in Boston over dinner when Dredze started musing out loud about Twitter.
Neither Dredze nor Paul had used Twitter at that point. But they had heard enough to be curious about the platform’s potential as a data pool. Just four years old, Twitter had already drawn more than 30 million users. (Today, that number is more than 300 million.)
More important than those numbers, however, was the way Twitter operated. On older platforms, such as Facebook and LinkedIn, users post messages that are directed inward, toward a circumscribed, semiprivate network of friends or connections. Tweets don’t work that way. They mostly face outward, toward the general public, leaving pretty much everything that happens on the platform right out there in plain view. Better yet, the company makes it easy for researchers to dig into that public-facing data by offering generous access to an array of application programming interface tools.
Given his interest in public health, Dredze steered the small talk over dinner in that direction: Do Twitter users talk online about their experiences with illnesses? What sorts of things do they say about their medications? Back in Baltimore a few days later, he sent an email to Paul reporting that he had run a quick query along those lines through a small sample of Twitter data and found “lots” of tweets containing the word “sick.”
“But of course,” that missive concluded, “it’s not such a simple problem.” He urged Paul to tackle that problem in an upcoming class project.
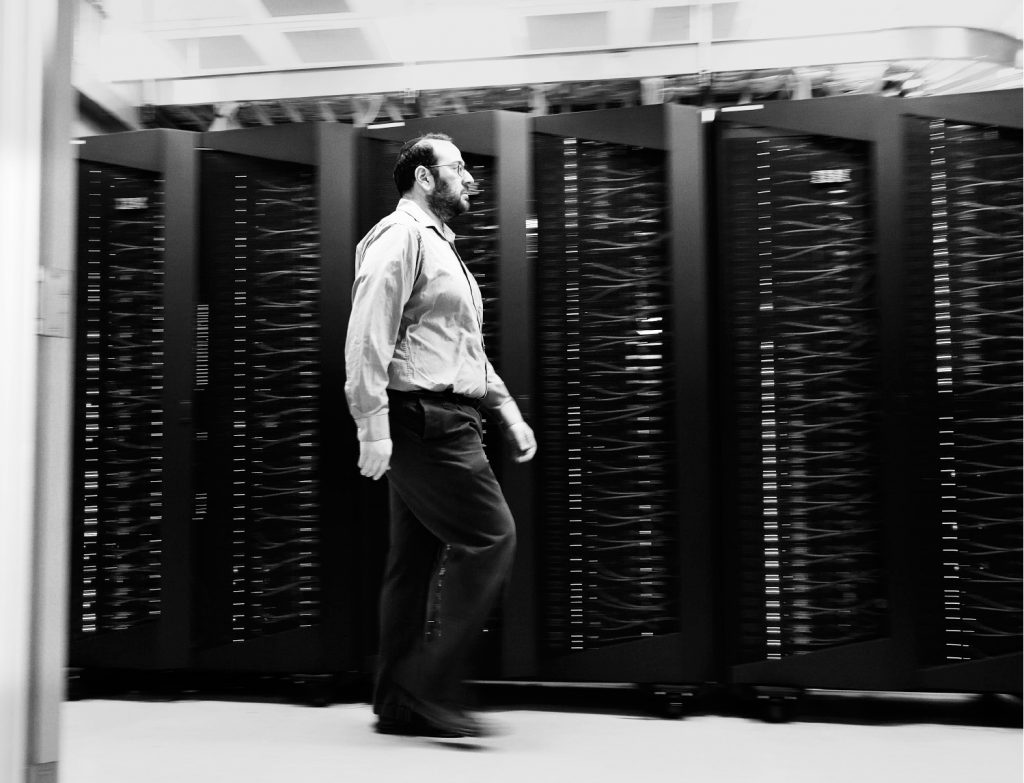
As a field, social monitoring has been growing up a at a breakneck pace—with Dredze at the fore.
The next year, 2011, Dredze and Paul published one of the early papers in social monitoring, mapping out a basic data landscape regarding which ailments are discussed most often on Twitter —the “winners” included influenza, allergies, and insomnia—and proposing a few ground rules for how researchers should approach them.
Some other pioneering researchers around the country had started finding their way into this new territory about the same time. The field in those early years had the aura of an old-fashioned gold rush, with scientists racing up various hills of data in willy-nilly fashion and staking claims to this, that, or another vein of potentially valuable information. There was little in the way of coordination and cooperation, especially across disciplinary lines.
“A lot of work was coming out of the computer science community, and a lot was coming out of the public health community, but there wasn’t a lot of cross-pollination,” Dredze says. “What we ended up with were computer science papers on health topics that sounded really interesting to computer scientists, but it turned out that the results weren’t actually very useful in public health.”
It worked the other way around as well. Public health experts would discover a vein of potentially valuable data, only to find computer scientists greeting their results with skepticism centered on the way they were employing various computational tools.
Dredze has shaped the course of his career to put himself in position to work effectively across the interdisciplinary boundaries of social monitoring. In addition to his main post in the Whiting School’s Department of Computer Science, he has affiliations at Johns Hopkins with the Malone Center for Engineering in Healthcare and the Bloomberg School of Public Health’s Center for Population Health Information Technology. He has a joint appointment in the School of Medicine’s Division of Health Sciences Informatics. He also did summer-long stints working on projects at Google, Microsoft, and IBM, and spent a recent sabbatical at Bloomberg LP.
Though still less than a decade old, social monitoring has been growing—and growing up—at a breakneck pace. The field’s coming of age is one of two primary themes in Dredze’s latest project, a book titled Social Monitoring for Public Health. Co-written with Paul (who is now an assistant professor of information science at the University of Colorado Boulder), it sorts through and synthesizes the findings of hundreds of social monitoring papers published over the last decade to present a clear picture of the current state of the science social monitoring.
CHARTING THE COURSE
The second theme in the book is more of a forward-looking affair. When the conversation in his tidy office on the third floor of Malone Hall takes a turn in this direction, Dredze leans in and shifts tone in a clear signal of his heightened interest.
“Can we make decisions about what social monitoring researchers should be focusing on and investing in over the next few years? I think we can.”
By a coincidence of timing, this conversation was sandwiched by a few weeks on either side between the publication dates of two papers looking at the recent drop in U.S. life expectancy rates—a December 2017 report by the U.S. Centers for Disease Control and Prevention and a February 2018 paper in the British Medical Journal. While still small, the drop is alarming because it comes on the heels of decades of steady, incremental gains—gains that most other developed countries in the world are continuing to see.
Taken together, the papers point to two main culprits as causes of the decline: fatal drug overdoses and suicides. That checks off two of the three boxes that make up Dredze’s answer to the question of where social monitoring is poised to make a big difference in the coming years. The third is gun violence.
None of these areas has been particularly well-studied so far by researchers in the field. To date, the health topic that has drawn the most interest is influenza. One reason the flu is such a popular topic is because it’s a convenient subject: There is a wealth of easily accessible online conversation around it, and results can be compared readily with rock-solid CDC data based on statistical monitoring of physician visits.
In Social Monitoring for Public Health, Dredze and Paul run through a lengthy list of the successful flu surveillance models developed to date by researchers looking at Twitter, Google searches, Wikipedia traffic, or various combinations of the three. One study even managed to track flu prevalence—successfully—via cancellation rates on OpenTable, a platform where customers make restaurant reservations.
All this work on the flu has put social monitoring in a position to help boost public health outcomes in at least three ways. First is a matter of speed. Researchers using web-based data sets can run models on a near real-time schedule, while the CDC’s reporting process, which runs through physicians’ offices, unfolds with a lag of two weeks.
Second, Dredze and Paul expect social monitoring researchers to continue to fine-tune their ability to zoom in on so-called fine-grained locations, boosting the reliability and detail of flu data at the level of cities, towns, and even neighborhoods so that residents and health professionals alike can get a more accurate sense for when to go on heightened alert.
Third, Dredze and Paul are hopeful about work under way that aims to move flu surveillance out of the real-time “nowcasting” mode and into accurately forecasting future trajectories.
“Showing where the flu is hitting today, compared with where it was two weeks ago—that can be a useful thing,” Paul says. “But if you can also look out there and see where it’s going to be next week or next month, that’s going to be even better.”
DAUNTING OBSTACLES
Compared with influenza, the work of social monitoring in those three areas Dredze flags as future priorities is just getting started. Looking out to the horizons of mental health, drug use, and gun violence, he sees a mix of urgent needs, daunting obstacles, and promising possibilities in every direction.
A key challenge in all three areas is the absence of baseline data. There is no central database in mental health where psychologists and psychiatrists are logging basic data on patient volume and diagnoses that then get toted up and distributed in the manner of CDC flu reports. The fact that many, and perhaps even most, cases of mental illness go undiagnosed, untreated, or both complicates matters even more.
The state of suicide statistics is illustrative here. The cause of 40,000 deaths a year in the United States, suicide has drawn quite a bit of attention from public health researchers in recent decades, but gaping holes remain in our basic knowledge of the phenomenon. Perhaps the most daunting: Experts can identify a very large group of people who exhibit risk factors, but they currently have no way of narrowing things down to identify who in that group will be among a comparatively small number of actual serious attempts and fatalities.
Even the best available statistics in suicide seem to come with important caveats. For example, a much-publicized 2015 study that confirmed high suicide rates among recent military veterans was based on data that stretched between the years of 2001 and 2009.
“How are you supposed to respond to increasing suicide rates when it takes somewhere around six, 10, or even 15 years just to confirm them in the first place?” Dredze asks.
Social monitoring researchers are starting the work of filling these holes. Recent studies cited in Social Monitoring for Public Health have identified social media data sets that are predictive of national suicide numbers and have developed ways to track the prevalence of known risk factors on Twitter. In China, researchers took a retrospective look at the social media activity of 130 suicide victims in search of measurable shifts in mood and activity in the lead-up to a suicide attempt.
Dredze teamed up with public health researcher John Ayers of the University of California, San Diego to look at online behavior around the 2017 Netflix television drama 13 Reasons Why. The series follows a teenager as he hunts through the remnants of a friend’s life in search of the reason she killed herself. Dredze was looking in particular for evidence of the Werther effect, a phenomenon named after an 18th-century European novel that became a pop culture phenomenon in its day and allegedly sparked a “contagion” of suicides among readers.
“We have decades of research that tells us that if you show someone’s suicide, it leads to increased suicide,” Dredze says. “Still, there was a debate back and forth about whether the show was having a positive influence or negative influence—that’s where our research steps in.”
The work showed sharp increases in searches for suicide topics—a jump of 34 percent on “teen suicide” and, alarmingly, 26 percent on “how to commit suicide.” (Previous social monitoring studies, Dredze notes, have demonstrated a link between increased search activity in these areas and increased suicide rates.) The news was not all bad, however: Searches for “suicide hotline” and “suicide prevention” rose by percentages that were nearly as large, indicating broad awareness about the availability of resources to help potential victims.
The other primary culprit singled out in those reports on declining U.S. life expectancy is drug overdoses. Fatal opioid overdoses, in particular, have jumped in recent years by annual percentage rates in the double digits.
“This is an all-hands-on-deck situation for public health professionals,” Dredze says. “And like with suicide, it’s another situation where it’s mostly happening outside of doctors’ offices, so this is also an area where online data have the potential to be really helpful.”
Paul points toward Reddit as one rich vein of data in this area, as users under cloak of anonymity feel freer to share their drug experiences. Paul is currently tracking Reddit conversations about a new product: highly concentrated marijuana oils. Drug users posting to Reddit have a tradition of informing readers how intoxicated they are by gauging highness on a scale of one to 10 in brackets at the end of posts, so Paul is looking to use that strange bit of social media etiquette to get an idea of the various potency levels of different oils.
“If you want to learn about something new going on with drugs, the internet is the place to be,” Paul says. “It’s where people go to have these conversations.”
The dearth of reliable data goes even deeper in Dredze’s third priority area, gun violence. Here, the situation is aggravated by a highly charged political environment that leaves politicians and bureaucrats skittish when it comes to funding even the most basic sorts of research. CDC health surveys, for instance, ask a series of questions about mental illness—whether respondents feel depressed, anxious, or suicidal—but nothing about guns.
“When it comes to really obvious questions around guns, we don’t have any answers yet,” Dredze says. “How many people use gun locks? How many people store their weapons in safes?”
Social monitoring is the fastest and cheapest way to start closing this data gap, Dredze adds. In recent years, researchers have used online news sources to develop a new database of gun violence incidents, gauged Twitter when it comes to predicting the outcome of polling questions on guns, and examined social media and search engine data to learn about public reactions in the wake of mass shootings.
“If I’m a scientist and I want to come up with policies that reduce gun violence, the place I start is the data—what do they say?” Dredze says. “They don’t say much of anything right now because there are very few data. This is definitely another area where I think we can do a lot of good work.”
#ETHICAL ISSUES
Social monitoring research involves constant interplay among three different fields: computer science, public health, and social media businesses. Each has developed its own sets of rules and limitations when it comes to conducting research.
Consider the case of a controversial 2014 study in which Facebook worked in tandem with academic researchers to measure whether the emotional tone of posts in a news feed can affect the mood of users. The project involved the active manipulation of news feed content to see how it altered people’s emotional state.
In academic medicine, any work along those lines would require approval from an institutional review board, not to mention explicit consent from study participants on a level much more specific than the all encompassing “terms of service” agreements that govern research as social media companies.
“We have to be very careful in our interactions here,” Dredze says. “We have to really think things through and anticipate what might be coming up down the road.”
It’s one thing if researchers can look over a public Twitter feed and identify someone as a fan of a certain band, he says, but it’s quite another when they can identify more sensitive information, such as someone being at risk for an anxiety disorder.
“Once we are doing that type of analysis, people have a right to become very wary about how this technology is being used,” Dredze says. “We need to be thinking through these ethical issues now and talking about how we’re maintaining data, sharing data, and using data.”
This article originally appeared in the 2018 Summer edition of the JHU Engineering Magazine