Johns Hopkins radiologists have found that a deep learning algorithm to detect tuberculosis (TB) in chest X-rays could be useful for identifying lung abnormalities related to COVID-19. These findings, published online in the Journal of Thoracic Imaging, suggest that deep learning systems could potentially assist clinicians in triaging and treating these high-risk patients, as well as help overcome the paucity of COVID-19 images available for machine learning development.
Chest X-rays have been proposed as a potentially useful tool for assessing COVID-19 patients, especially in overwhelmed emergency departments and urgent care centers.
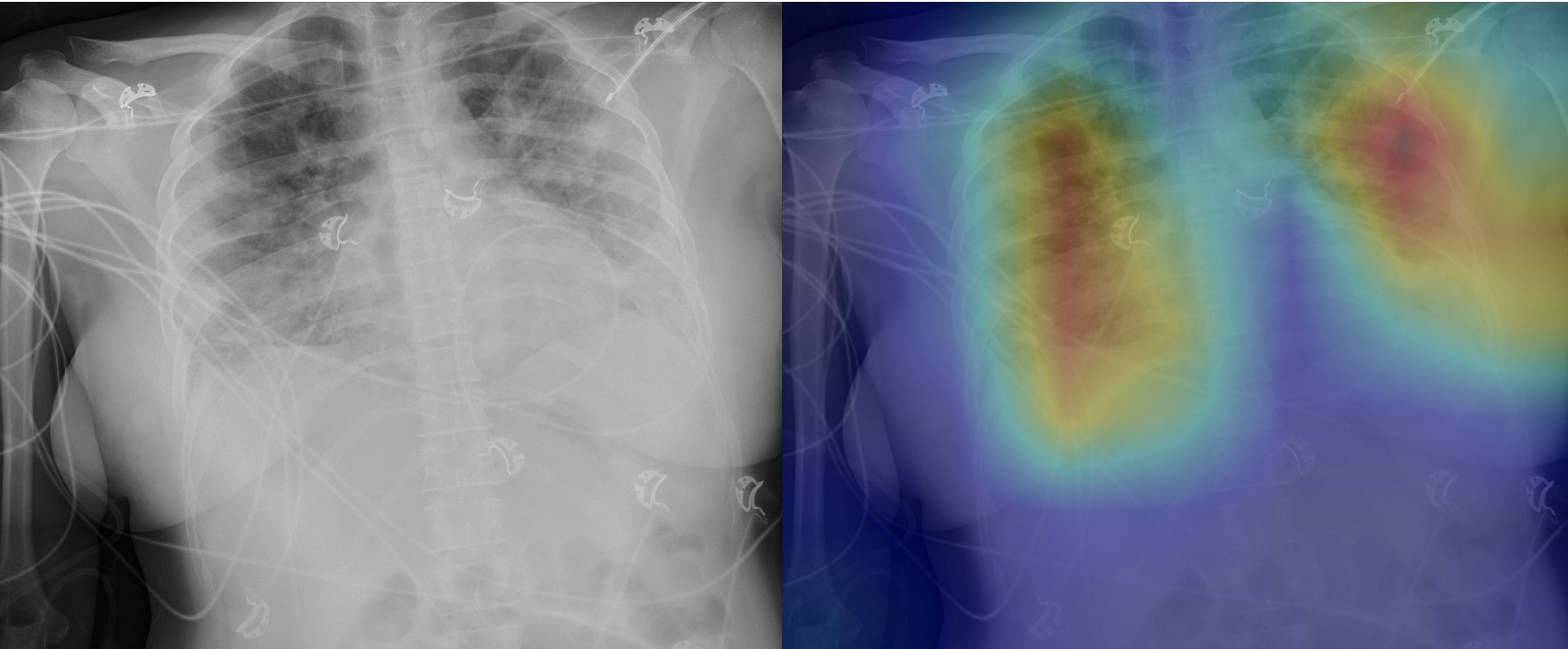
Algorithm localizing COVID-19 pneumonia on chest x-ray
The study was based on the observation that chest X-ray abnormalities from COVID-19 appear very similar to those of TB patients. The research team hypothesized that a deep learning model already trained to identify TB in X-rays would also work well to identify signs of the novel coronavirus.
“We found good generalization of our TB model toward COVID-19,” says radiology resident Paul Yi, co-director of the Radiology AI Lab and affiliate faculty of the Malone Center for Engineering in Healthcare. “Our goal was to demonstrate the ability of a deep learning model that had never ‘seen’ a case of COVID-19 to identify these cases. Because COVID-19 is a new infection, large datasets are not currently available to train deep learning models. We hypothesized that images of other infections with similar appearances to COVID-19 could be used to train models capable of identifying this new disease.”
Yi, along with co-authors Tae Kyung Kim, a medical student at Johns Hopkins School of Medicine, and Cheng Ting Lin, director of Thoracic Radiology in the Russell H. Morgan Department of Radiology and Radiological Science, collected 88 publicly available frontal chest X-rays from patients with confirmed COVID-19 diagnoses. Of the 88, the model correctly classified 78 of them as “positive” for COVID-19, for an 89 percent success rate.
While these results are encouraging, the team acknowledges their study’s limitations. For example, although the model can identify COVID-19 positive x-rays, it may not be able to distinguish it from other diseases that cause similar lung abnormalities.
Still, further research into this area is certainly warranted as the pandemic continues. “These results are a proof-of-concept for a potential new screening method for COVID-19 in clinical settings,” adds Yi. “Deep learning models could facilitate faster triage in emergency departments, point-of-care interpretation for non-radiologists on the frontline, and potential workload reduction for radiologists.”