Much is known about schizophrenia, from its hereditary component to the fact that its characteristic hallucinations and delusions are linked to alterations in brain activity.
Less is known about the causes of this disease that affects 3 million Americans, however. Researchers posit that changes in brain structure and chemistry are involved, and data provided by analyses of genetic mutations and through brain imaging is yielding important clues, but a complete picture has proved elusive.
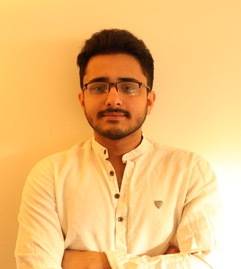
Sayan Ghosal, a doctoral candidate in Electrical and Computer Engineering at the Whiting School of Engineering, is working to change that. His approach, a model called Genetic and Multimodal Imaging data using Neural-network Designs, “G-MIND,” combines data from both imaging and genetics to provide a better understanding of biomarkers associated with schizophrenia—research that could not only help predict who is at risk of developing the disease but also lead to more effective treatments.
“We hope this model can be used to capture interactions between brain activity and genetic mutations that are associated with schizophrenia,” Ghosal says. “Our model has the ability to fuse all these different views into a single framework and jointly learn different representative patterns that are predictive of schizophrenia. However, fusing different data methods is hard, so we also had to develop our own algorithms that exploit the structure shared between the data modalities to find a solution.”
Ghosal’s model starts with analyzing genetic mutations in the form of single nucleotide polymorphism, or SNP, data, which contains information about alterations in the DNA structure. It helps identify locations in the DNA that contain predictive information about schizophrenia, essentially providing an “importance map” that tells researchers which regions of DNA contain potential schizophrenia “landmarks.” This information can help researchers focus on specific regions of the DNA, saving them from scouring the whole genome.
Assistant Professor Archana Venkataraman says this approach stands out from others because it combines the two main viewpoints of neuropsychiatric disorders: behavioral and cognitive dysfunction is caused by altered brain activity, and it is highly heritable.
“Most studies focus on one viewpoint or the other, which provides an incomplete picture of the underlying causes and mechanisms,” says Venkataraman, the principal investigator of the Neural Systems Analysis Lab of which Ghosal is a member. “Sayan’s work attempts to bridge these two domains. He has developed a deep neural network that can extract predictive biomarkers of brain function and genetic variation, while also providing diagnostic information. Ultimately, his work takes a crucial step toward a more complete and personalized characterization of neuropsychiatric disorders, which can be used to design targeted therapeutics.”
Ghosal’s paper about the research, “G-MIND: An End-to-End Multimodal Imaging-Genetics Framework for Biomarker Identification and Disease Classification” won the Best Student Paper Award at the virtual Image Processing Conference of SPIE Medical Imaging in February.
“Receiving the award was definitely exciting, and it also feels good to see that our modeling strategy and our approach excite other prominent researchers,” he says.
Looking ahead, Ghosal wants to expand this predictive research to improve understanding of how the genetic mutations associated with schizophrenia alter the biological pathways leading to the often devastating disease.
“It’s extremely difficult to localize the reasons behind disorders like schizophrenia,” he says. “Performing pharmacological and biochemical techniques on human subjects, which is the current norm for treating those suffering from schizophrenia, is very complicated. Building noninvasive, data-driven techniques to uncover the underlying causes of the disease is needed now more than ever.”
-Story by Wick Eisenberg